Decoding Speech from Brain Waves: New Frontiers in AI
Written on
Chapter 1: Introduction to AI and Brain-Computer Interfaces
Recent advancements in artificial intelligence have led to the development of innovative methods to decode speech from brain activity. This technology holds great potential for individuals with speech impairments, enabling them to communicate more effectively.
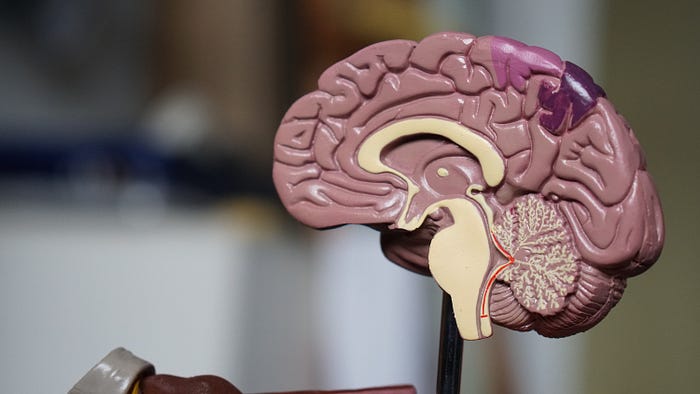
Chapter 2: The Challenge of Communication Loss
Every year, countless individuals experience a loss of communication abilities due to accidents, strokes, or degenerative conditions. The United Nations estimates that over 1 billion people worldwide live with some form of disability. To address these challenges, brain-computer interfaces (BCIs) have emerged as critical tools, allowing individuals with speech paralysis to communicate at a rate of approximately 15 words per minute.
Section 2.1: Limitations of Invasive Interfaces
Traditional BCIs often require surgical implantation of electrodes into the brain, which poses significant risks, including infection and rejection. This has led researchers to explore non-invasive alternatives for decoding language.
Section 2.2: Non-Invasive Techniques
Two primary techniques have been proposed for this purpose:
- Magnetoencephalography (MEG): A functional neuroimaging method that maps brain activity using magnetic fields.
- Electroencephalography (EEG): A diagnostic test that records electrical activity in the brain via electrodes.
Despite advancements, these methods can produce noisy data that varies widely between individuals. Consequently, researchers often prefer to extract specific features from the raw signals rather than using them directly.
Chapter 3: Recent Advances in Speech Decoding
A recent study aimed to create a model capable of decoding spoken words from brain recordings. However, a significant challenge remains: the precise representation of spoken language in the brain is still not fully understood.
Beyond the Hype: Unraveling AI Myths, Realities, & Governance - This video dives into common misconceptions about AI and explores its real-world implications and governance challenges.
The researchers began their work with healthy subjects who listened to recordings in their native language. They proposed a novel approach using a contrastive loss function, originally designed to align text and image representations.
Section 3.1: Building a New Brain Module
The researchers developed a brain module tailored for analyzing spectrograms, utilizing a spatial attention layer and convolutional layers. This module aimed to maximize alignment between the representations of sound and brain signals.
724: Decoding Speech from Raw Brain Activity — with Dr. David Moses - Dr. Moses discusses the intricacies of decoding speech from brain signals and shares insights on the future of this research.
To train the model, the authors compiled a dataset of MEG and EEG recordings from participants listening to short stories. The model's performance was evaluated against 1,500 segments of brain recordings.
Chapter 4: Results and Interpretations
The outcomes demonstrated that MEG outperformed EEG in decoding tasks. The model's ability to identify exact audio segments was noteworthy, and performance improved when considering likely segments.
Section 4.1: Understanding Model Performance
The researchers also conducted analyses to determine the model's influential factors. They found that higher-level representations, such as part-of-speech and phrase embeddings, significantly impacted predictions, suggesting the model relies more on semantic and syntactic understanding rather than on individual word representations.
Parting Thoughts
This innovative model represents a significant step forward in identifying speech segments from brain activity, a noteworthy feat given the inherent noise in the data. The development of a streamlined, end-to-end architecture has simplified the analysis process compared to traditional methods.
While the research shows promise, there remains work to be done before this technology can be applied clinically. Future iterations will need to refine the model's understanding of more complex language structures.
What do you think? Share your thoughts in the comments.
If you found this topic intriguing, feel free to explore my other articles, connect with me on LinkedIn, or check out my GitHub repository for resources related to machine learning and AI: